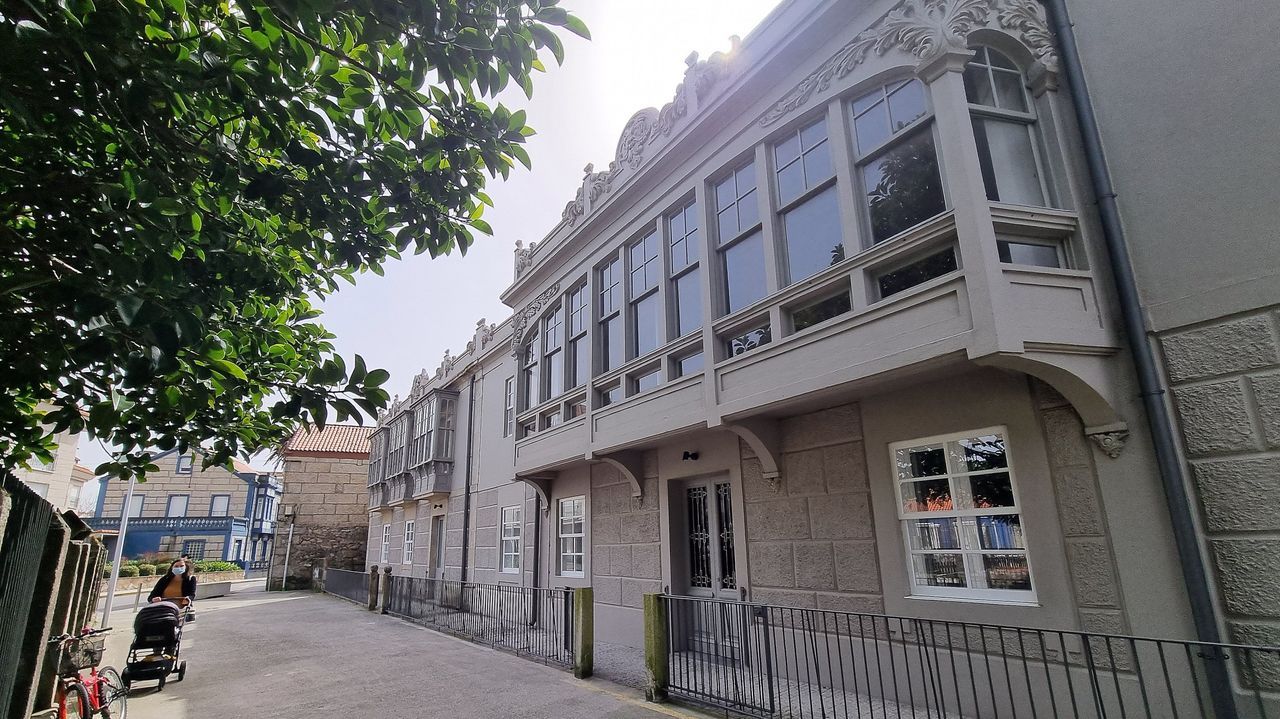
The role of traditional language in automated speech attention.
Introduction
Voice popularity has revolutionized the way we engage with technology. From digital assistants like Siri and Alexa to automated transcription approaches, using traditional language has been instrumental in convalescing the accuracy and reliability of those applied sciences. In this article, we will delve into the role of usual language in automatic speech recognition, exploring its elements, functions, demanding situations, and the long term of this exciting intersection.
What is automated speech awareness?
Definition and fundamentals
Automatic speech acceptance (AVR) refers back to the ability of a computing device equipment to determine and know spoken phrases. This method consists of a number of steps, along with:
History of speech recognition
The Website link first experiments with voice attention date again to the Fifties. However, it used to be within the 1990s that extra evolved procedures all started to be developed, driven via improvements in hardware and device.
The position of natural and organic language in automated speech recognition
Definition of normal language
Natural language refers back to the language that we use day by day to keep up a correspondence with each one different. Unlike synthetic or formal language, that is full of nuances, idiomatic expressions and dialect modifications.
Interaction among RAV and NLP
Combining RAV with NLP suggestions allows for machines to interpret now not simply what is free speech to text referred to, yet also how it really is reported. This carries sides along with intonation, accents and cultural contexts.
Key add-ons of computerized speech recognition
1. Acoustic sign processors
These systems study the acoustic qualities of speech, which includes frequency and amplitude.
2. Phonetic models
Phonetic versions aid wreck down spoken phrases into exceptional sounds or "phonemes."
three. Statistical algorithms
They use possibilities to are expecting what note is being suggested situated on outdated contexts.
four. Linguistic databases
Databases furnish a framework for the way sentences are established and what combos are so much possibly depending on context.
Practical purposes of computerized speech recognition
Virtual assistants
Virtual assistants are maybe the such a lot visible utility of RAV today. They permit users to perform initiatives the use of sensible verbal instructions.
Automatic transcription
In skilled settings, resembling conferences or meetings, RAV tactics can generate wireless transcriptions.
Current challenges in automated speech recognition
Acoustic variability
Variability in accents, tones, or even ambient noise can make RAV tricky.
Linguistic ambiguity
Words could have distinct meanings based on the context; This calls for in-intensity research by way of the NLP.
How does device gaining knowledge of boost RAV?
Machine mastering (ML) performs a needed function in bettering RAV systems with the aid of letting them invariably study from new audio samples and user interactions.
The future of automatic speech recognition
It is envisioned that with long run technological advances, resembling deep neural networks and developed synthetic intelligence, we are going to succeed in a factor the place interaction with machines is almost indistinguishable from a wide-spread human dialog.
FAQ (Frequently Asked Questions)
What technologies are used in computerized speech consciousness?
Main technology consist of virtual sign processing (DSP), deep neural networks (DNN), hidden-Markov types (HMM), and improved statistical algorithms.
Is automatic speech popularity exact?
Accuracy is dependent widely at the context and acoustic high-quality; However, many leading-edge methods attain accuracies extra than 95% lower than most efficient conditions.
How does the accessory influence the RAV?
Accents can significantly have effects on accuracy; However, the units are invariably enhancing to evolve to numerous dialect patterns.
What is the change among RAV and NLP?
While RAV focuses often on audio-textual content conversion, NLP speech typing works on that textual content to take into account its that means and aim in the back of it.
Can they take into account the several languages?
Yes, many systems are designed to understand assorted languages; However, its effectiveness would differ based on each detailed language by means of its structural complexity.
What long term does this expertise have?
With persisted advances in synthetic intelligence and system gaining knowledge of, human interplay with machines is anticipated to grow to be even greater intuitive and fluid.
Conclusion
In precis, we will verify that the function of normal language in automated speech recognition is fundamental to go in direction of more productive communication between human beings and machines. The efficient blend among these two disciplines grants to revolutionize our every day interactions with expertise. As we proceed to innovate and boost those techniques, it is going to be intriguing to work out how our future verbal exchange abilities evolve.